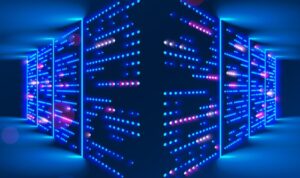
(Andrey Suslov/Shutterstock)
Pretrained large language models (LLMs) like GPT-4 and Gemini are great, but real competitive advantage comes from combining LLMs with private data. Unfortunately, there are questions sa to how well companies have prepared their private data estates for GenAI, according to a new report from MIT Technology Review.
There’s no doubt that generative AI has caught the attention of organizations, who are eager to use LLMs to build chatbots, copilots, and other types of applications. Scaling AI or GenAI is a “top priority” for 82% of the executives surveyed for MIT Technology Review’s report, which is titled “AI readiness for C-Suite leaders” and was conducted on behalf of ETL vendor Fivetran.
And organizations have a good idea what data they want to use with GenAI, according to the survey, which found 83% of organizations have already identified sources of data to use for AI or GenAI.
But how well are organizations prepared to actually connect the dots on GenAI and deliver the data to GenAI applications when it’s needed, where it’s needed, sufficiently cleaned and prepped, and in the proper format? And to do all that without putting privacy or security in jeopardy?
That is the real trick, of course, and it is something that not a lot of organizations are great at–at least not yet.
The difficulties in getting all your data tools and techniques onto the same pages are immense. As IDC analyst Stewart Bond notes, a recent IDC study concluded that the average organization has “over a dozen different technologies just to harvest all the intelligence about their data and the same number to integrate, transform, and replicate it,” he tells MIT Tech Review. “The technical debt out there is very real.”
Older data integration and ETL tools developed for centralized data warehousing initiatives may not fit the bill for new GenAI use cases, MIT Tech Review says in its report. That’s why it’s notable that the survey found that 82% of surveyed tech execs say they “are prioritizing acquiring data integration and data movement solutions that will continue to work in the future, regardless of other changes to our data strategy and partners.”
Getting better data integration and ETL/data pipeline tools is clearly a priority, but there are other important investments to make, the report found. While 64% of survey takers say data integration and ETL/pipeline tools are one of their top two GenAI investment priorities, 35% cited data lakes as a priority item, while 31% cited data transformation tools. Data catalogs and LLM investments, meanwhile, tallied just 7% shares, with vector databases and computational layers in the middle.
Tech executives surveyed identified numerous challenges in building that data foundation, including data integration and building data pipelines; data governance and security; and data quality, among other issues (see figure).
The top four tasks that organizations struggle with the most on the data integration/data pipeline front include: managing data volume; moving data from on-premises to the cloud; enabling real-time access; and managing changes to data. Integrating data from different geographies and integrating third-party data also garnered significant responses, according to the study.
Fivetran CEO George Fraser, a 2023 Datanami Person to Watch, concurs that a strong data foundation is a requirement for GenAI success.
“You want to make sure that you have an enterprise data warehouse with clean, curated data, which should be supporting all of your traditional BI and analytics workloads, before you go and start hiring a lot of data scientists and initiating a lot of generative AI projects,” Fraser says in the report. “If organizations don’t start by building strong data foundations, their data scientists will squander their time on basic data integration and cleanup.”
The survey data becomes a bit more nuanced when it comes to the data governance, compliance, and reporting side of the equation.
While large percentages of survey respondents indicated that their biggest challenges to preparing data for AI was data governance and security (cited by 44% of respondents) and data integration or pipelines (cited by 45%), a deeper examination of the data reveals a meaningful split.
Namely, the survey shows that positive concerns about security and governance were highly focused among government and financial services institutions–two highly conservative sectors–while tech execs in manufacturing, retail, and other industries did not share those same security and governance concerns at nearly the same rate.
“Organizations may have no control over someone using a piece of data in a business application and sending it to a generative AI model,” IDC’s Bond said in the report. “These are critical concerns.”
You can read the full report here.
Related Items:
Making the Leap From Data Governance to AI Governance
The Rise and Fall of Data Governance (Again)
Finding the Data Access Governance Sweet Spot