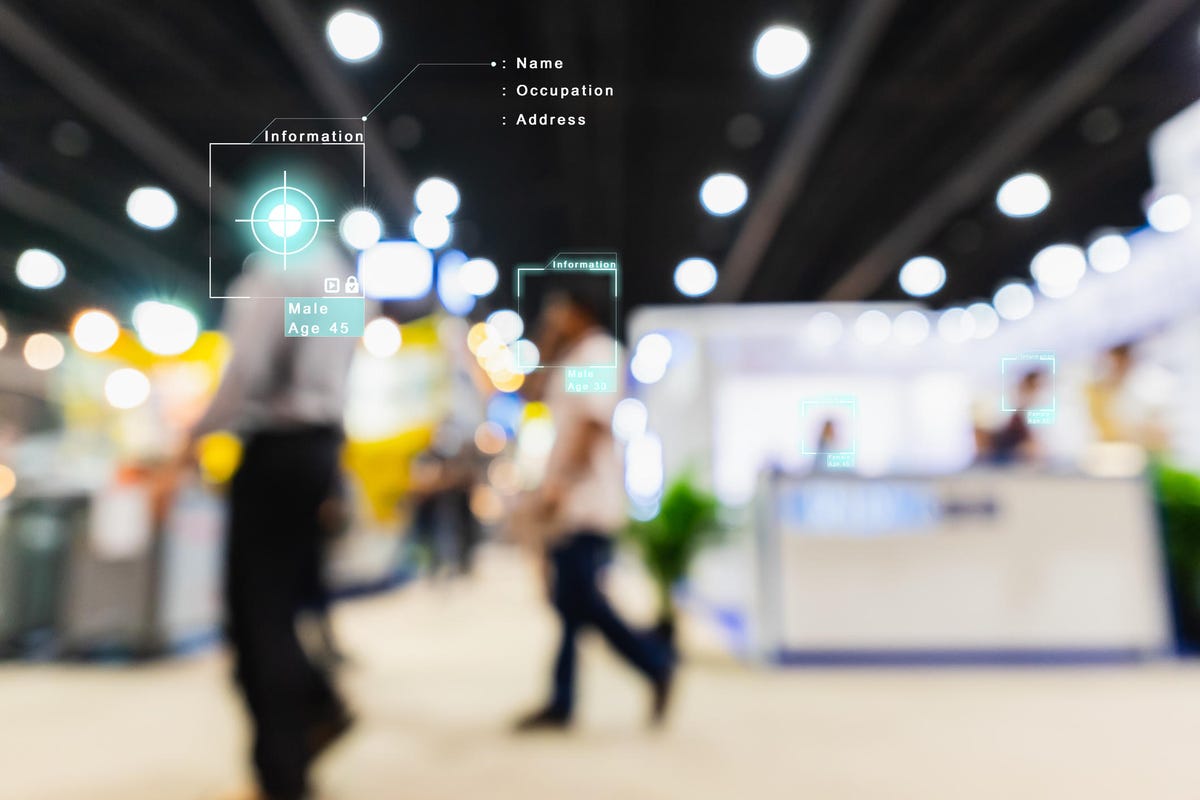
Although AI systems are becoming more sophisticated – and pervasive – by the day, there is currently no common stance on the best way to check algorithms for bias.
Image: Getty Images/iStockphotoWhen it comes to detecting bias in algorithms, researchers are trying to learn from the information security field – and particularly, from the bug bounty-hunting hackers who comb through software code to identify potential security vulnerabilities.
The parallels between the work of these security researchers and the hunt for possible flaws in AI models, in fact, is at the heart of the work carried out by Deborah Raji, a research fellow in algorithmic harms for the Mozilla Foundation.
Presenting the research she has been carrying out with advocacy group the Algorithmic Justice League (AJL) during the annual Mozilla Festival, Raji explained how along with her team, she has been studying bug bounty programs to see how they could be applied to the detection of a different type of nuisance: algorithmic bias.
SEE: An IT pro’s guide to robotic process automation (free PDF) (TechRepublic)
Bug bounties, which reward hackers for discovering vulnerabilities in software code before malicious actors exploit them, have become an integral part of the information security field. Major companies such as Google, Facebook or Microsoft now all run bug bounty programs; the number of these hackers is multiplying, and so are the financial rewards that corporations are ready to pay to fix software problems before malicious hackers find them.
“When you release software, and there is some kind of vulnerability that makes the software liable to hacking, the information security community has developed a bunch of different tools that they can use to hunt for these bugs,” Raji tells ZDNet. “Those are concerns that we can see parallels to with respect to bias issues in algorithms.”
As part of a project called CRASH (the Community Reporting of Algorithmic System Harms), Raji has been looking at the ways that bug bounties work in the information security field, to see if and how the same model could apply to bias detection in AI.
Although AI systems are becoming more sophisticated – and pervasive – by the day, there is currently no common stance on the best way to check algorithms for bias. The potentially devastating effects of flawed AI models, so far, has only been revealed by specialized organizations or independent experts, with no connection to one another.
Examples include Privacy International digging out the details of the algorithms driving the investigations led by the Department for Work and Pensions (DWP) against suspected fraudsters, to MIT and Stanford researchers finding skin-type and gender biases in commercially released facial-recognition technologies.
“Right now, a lot of audits are coming from different disciplinary communities,” says Raji. “One of the goals of the project is to see how we can come up with resources to get people on some sort of level-playing field so they can engage. When people start participating in bug bounties, for example, they get plugged into a community of people interested in the same thing.”
The parallel between bug bounty programs and bias detection in AI is evident. But as they dug further, Raji and her team soon found that defining the rules and standards of discovering algorithmic harms might be a bigger challenge than establishing what constitutes a software bug.
The very first question that the project raises, that of defining algorithmic harm, already comes with multiple answers. Harm is intrinsically linked to individuals – who in turn, might have a very different perspective from that of the companies designing AI systems.
And even if a definition, and possibly a hierarchy, of algorithmic harms were to be established, there remains an entire methodology for bias detection that is yet to be created.
In the decades since the first bug bounty program was launched (by browser pioneer Netscape in 1995), the field has had the time to develop protocols, standards and rules, that ensure that bug detection remains beneficial to all parties. For example, one of the best-known bug bounty platforms, HackerOne, has a set of clear guidelines surrounding the disclosure of a vulnerability, which include submitting confidential reports to the targeted company and allowing sufficient time to publish a remediation.
Raji has been looking at the ways that bug bounties work in the information security field, to see if and how the same model could apply to bias detection in AI.
Image: Deborah Raji“Of course, they’ve had decades to develop a regulatory environment,” says Raji. “But a lot of their processes are a lot more mature than the current algorithmic auditing space, where people will write an article or a Tweet, and it’ll go viral.”
“If we had a harms discovery process that, like in the security community, was very robust, structured and formalized, with a clear way of prioritizing different harms, making the whole process visible to companies and the public, that would definitely help the community gain credibility – and in the eyes of companies as well,” she continues.
Corporations are spending millions on bug bounty programs. Last year, for instance, Google paid a record $6.7 million in rewards to 662 security researchers who submitted vulnerability reports.
But in the AI ethics space, the dynamic is radically different; according to Raji, this is due to a misalignment of interests between AI researchers and corporations. Digging out algorithmic bias, after all, could easily lead to having to redesign the entire engineering process behind a product, or even taking the product off the market altogether.
SEE: The algorithms are watching us, but who is watching the algorithms?
Raji remembers auditing Amazon’s facial recognition software Rekognition, in a study that concluded that the technology exhibited gender and racial bias. “It was a huge battle, they were incredibly hostile and defensive in their response,” she says.
In many cases, says Raji, the population affected by algorithmic bias are not paying customers – meaning that, unlike in the information security space, there is little incentive for companies to mend their ways if a flaw is found.
While one option would be to trust companies to invest in the space out of a self-imposed willingness to carry out ethical technology, Raji isn’t all that confident. A more promising avenue would be to exert external pressure on corporations, in the form of regulation – but also thanks to public opinion.
Will fear of reputational damage unlock the possibility of future AI-bias bounty programs? For Raji, the answer is evident. “I think that cooperation is only going to happen through regulation or extreme public pressure,” she says.